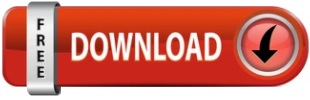
- #Use imagej software for grain map how to
- #Use imagej software for grain map manual
- #Use imagej software for grain map mac
Our results demonstrate that automatic computer vision enables a systematic, fast, repeatable method of grain size analysis, across large data sets, improving the accuracy of experimentally determined grain growth kinetics. This additional population of grains, when fit to the normal grain growth law, highlights the influence of improved accuracy and sample size on the estimation of grain growth kinetic parameters.
#Use imagej software for grain map manual
Using this automated approach, we have been able to identify a significant proportion of small grains, which have been overlooked when using manual methods.
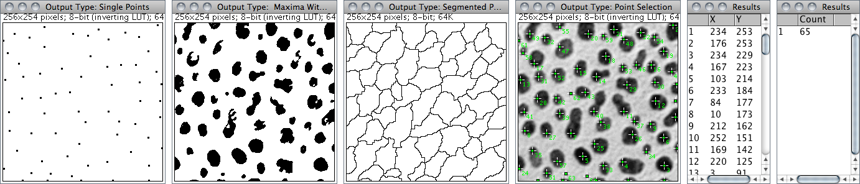
To accurately measure the sizes of these small grains, we have developed a computer vision workflow using a watershed transformation, which rapidly measures 68% more grains and produces a 20% improvement in the average grain size accuracy and repeatability when compared with manual methods. The grain growth dynamics of spinelorthopyroxene mixtures in the upper mantle are modeled here by experimentally producing small grain sizes in the range of 0.5 to 2 µm radius at pressures and temperatures equivalent to the spinel lherzolite stability field. The experimentally determined grain sizes can be fit to the normal grain growth law ( G n – G 0 n) = k 0 t∙exp(–Δ H/R T) and then be used to determine grain size throughout the mantle and geological time. Experimental studies provide an opportunity to simulate the grain growth kinetics of mantle aggregates. The intercept method, using only a single test circle (rather than the three concentric test circles recommended in E 112) yielded an estimate of the grain size of 6.76 for both the 9 highest intercept counts with 42 intersected grains, and for the 23 highest intercept counts with >20 intersected grains per test circle application. The decision whether the point is on a boundary or not is made by evaluating that pixels and its neighborhoods properties by using a fuzzy system.
#Use imagej software for grain map mac
If you are really interested in this topic ImageJ was actually developed from NIH-Image (USA) (Apple mac based software)and the available literature on that software and on image analysis in general was superb.Earth’s physical properties and mantle dynamics are strongly dependent on mantle grain size, shape, and orientation, but these characteristics are poorly constrained. The fuzzy logic grain boundary detection algorithm (FLGBD) developed in this paper traces the entire image, pixel by pixel, and detects the pixels that belong to a boundary. Unless you pay for expensive custom software, you need to do some reading round and a bit of practice, before you will get the best results from actual micrographs. Here's a link (not that detailed) which sumarises the basic steps in using imageJ for grain size analysis. In image J you use the straight line tool to retrace the length of the micron bar, then use the set scale function. On your micrograph (or a reference one taken at the same magnification) you need a length scale (colloquially called a micron bar). Tango For generating a wide variety of maps, e.g.
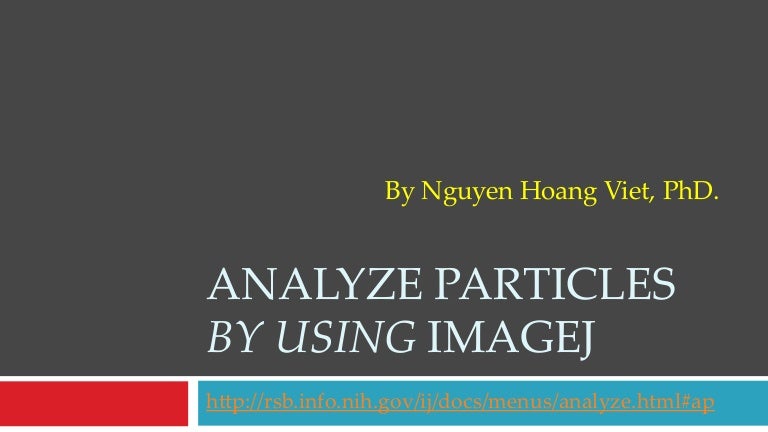
To make real world measurements your image needs to be calibrated. We use Zeiss Smartsem software for controlling the microscope. His son used to run a website and do technical support for image analysis software that John wrote as a set of IA plugins for Photoshop.
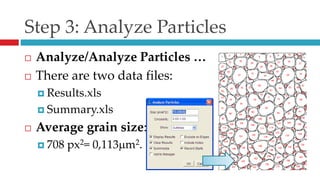
I would recommend having a look at "The Image Processing and Analysis Cookbook" by John C Russ.
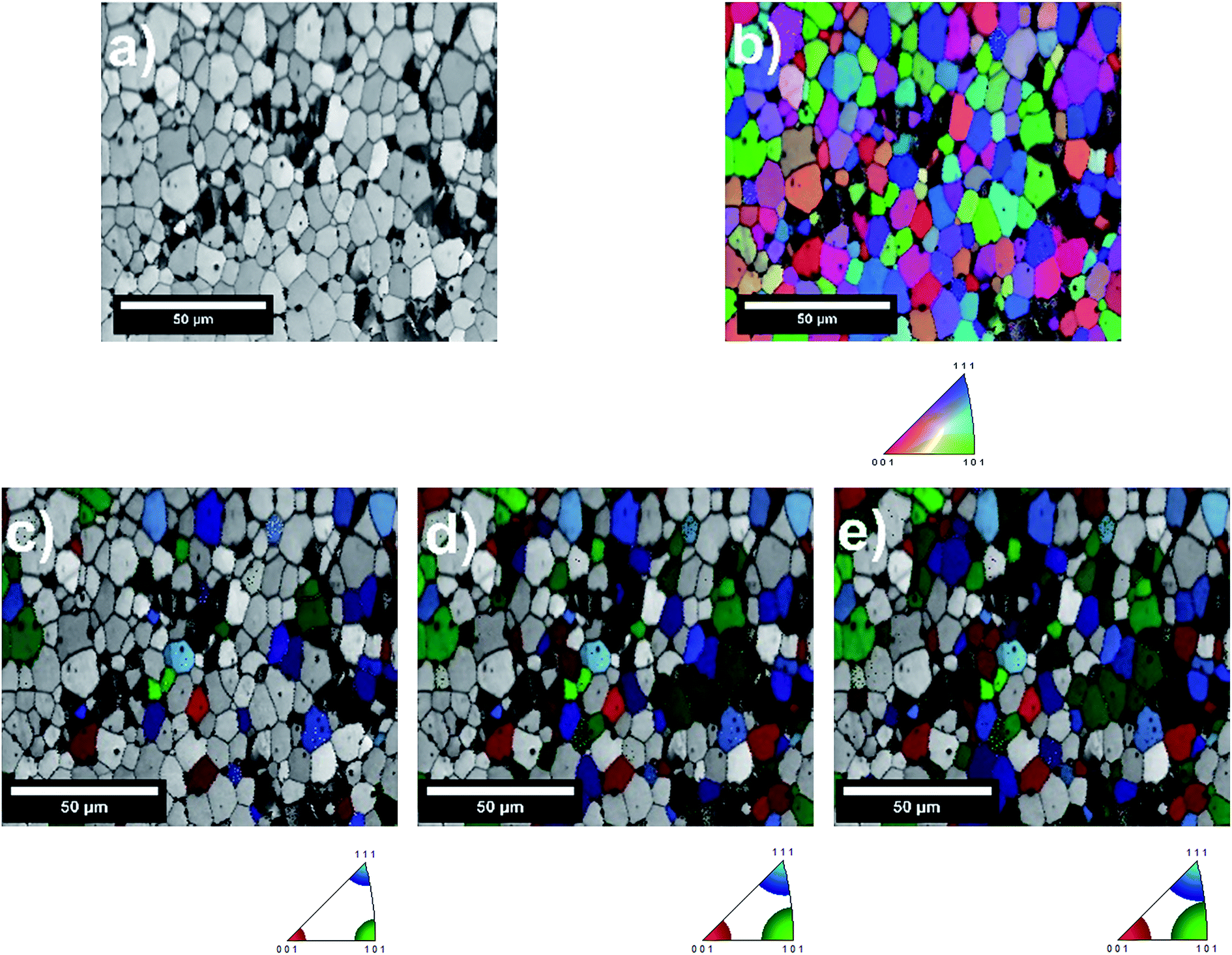
Once segmented (aka thresholded or binarised) the image might need pruning, dilatation erosion etc. you might need to use a specialised background leveling/subtraction process.
#Use imagej software for grain map how to
So normally however you have to do a bit (or a lot) of pre-processing, i.e. ImageJ tutorial, the terrible oneGrain size distribution analysis using ImageJ - Check how bad is this ideaIn this video, I am showing how to get histogram. The human eye tends to perform its own automatic localised background subtraction on such images, and images tend to be worse than what you think from a cursory visual inspection. the closer your original image is to say black lines (say the grain boundaries) on a 'flat' (virtually pure consistent) white background the better. This is where the quality of the original image is key. Thresholding essentially is a process where you 'segment' the actual grey levels of the pixels in the image into either black or white, (you tend to do this manually using a slider bar, whilst observing a preview window showing the resulting binary image, some software suggests what it thinks is a reasonable slider bar position automatically). Typically (unless in colour) your raw image will have a range of grey levels, but this kind of software essentially wants (eventually) what is called a binary image, i.e.
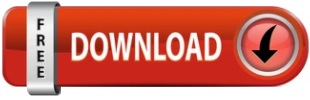